2021. 6. 10. 11:26ㆍalgorithm/Deep Learning
출처 : https://www.google.co.kr/amp/s/blog.griddynamics.com/customer2vec-representation-learning-and-automl-for-customer-analytics-and-personalization/amp/
아래 글은 해당 블로그의 요약글이며, 상세한 내용은 상기 출처를 참고 바랍니다.
———————————————————————————————————-
Personalization과 추천 알고리즘 맥락에서 word2vec 방법론의 활용
- 더 정확한 예측력
- 더 유연한 모델 아키텍처
방법론상의 특징
- semantic representation (embeddings) : cluster and analyze embeddings to gain deep insight into semantics of customer behavior
- 다시 말하자면, tokens의 sequence 데이터를, 문장의 개념을 도입하여, context를 입히는 방식임
- 어떤 것을 임베딩하는지에 따라서 홍길동2vec 이라고 부르며
- 하나만 임베딩 하기도 하고, 여러 가지를 순서대로 혹은 섞어서 임베딩 하기도 한다 (여러 가지를 순서대로 임베딩하는 것을 downstream model이라고 이해해보자)
- 상품과 고객간의 유사성proximity를 측정하기 위한 임베딩 방식을 사용
딥러닝 기반 방법론과 기존 basic 방법론과의 차이 (기존 personalisation 방식의 한계 기반) ————————-
look-alike modeling
= 비슷한 타겟 오디언스를 찾기 위한 방법
방법론상 특징
1> regression or classification
2> hand-engineering - 데모.행동 데이터.타겟고객에 대한 라벨링(acquisition or retention)
(-) 과거 이력 정보를 활용한 트레이닝 (하단 그림 참조)
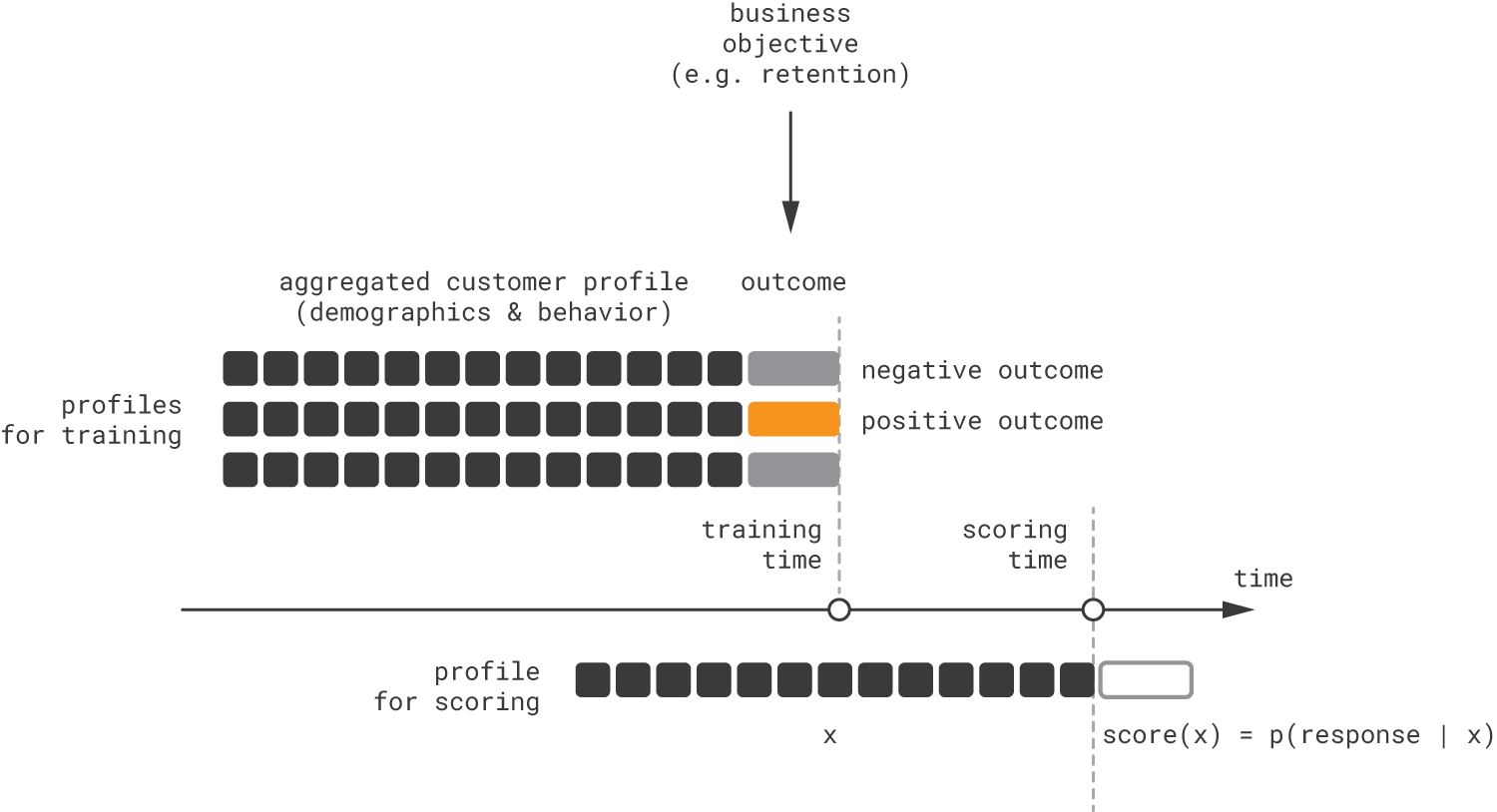
collaborative filtering
product recommendation 위해서는 고객 뿐 아니라 상품-고객 pair에 대한 예측(personalised context에서 상품 rank 만들기 위한 예측)
방법론상 특징
1> one-hot encoding
2> sparse feature에 대해 classification과 regression 하려다보니 위의 사례보다 dimensionality reduction이 필요
3> 다시 말하면, matrix completion problem 문제임 : matrix factorization 등
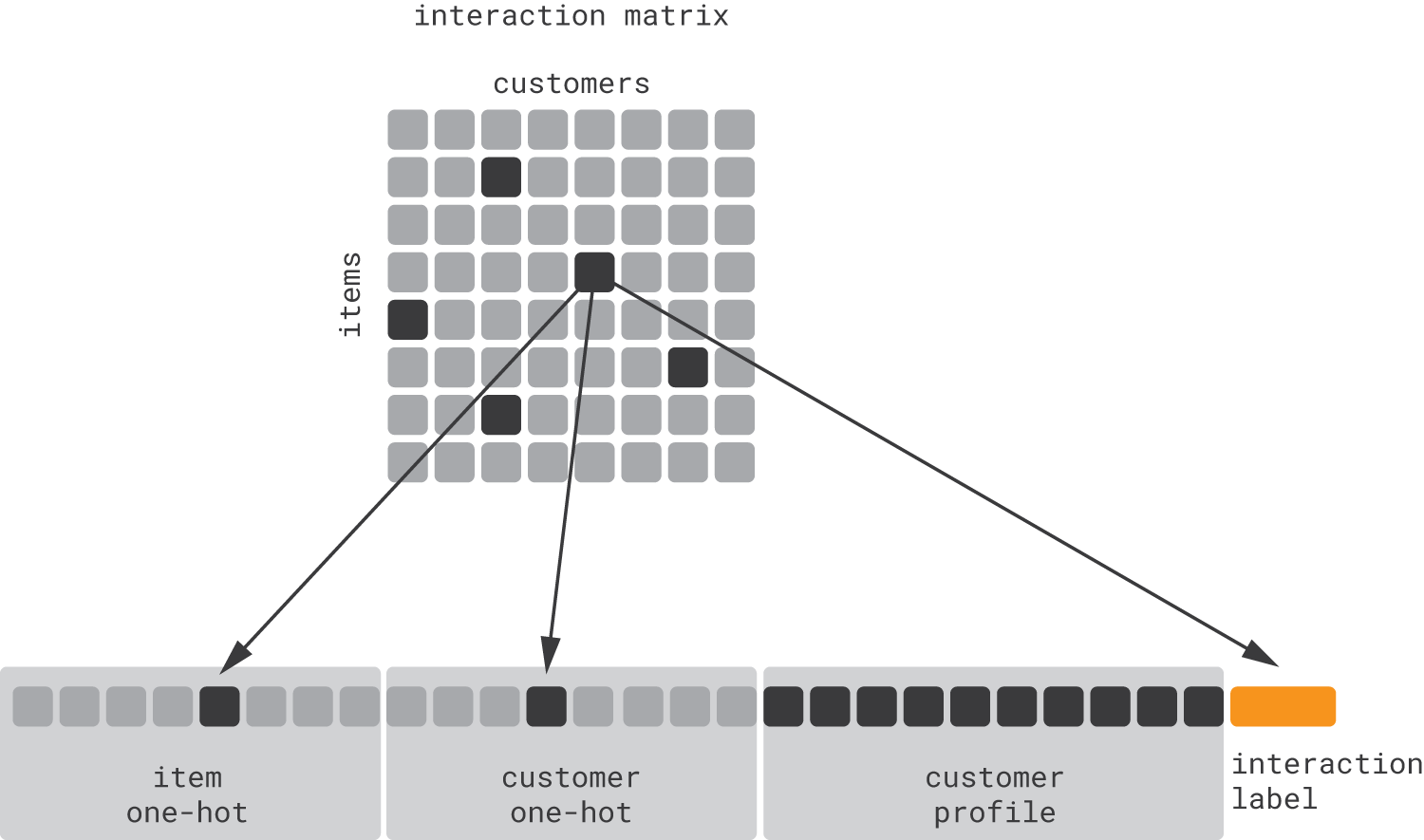
(-) 과거의 데이터 활용
(-) 아예 정보가 없는 고객/상품은 추천이 안 됨
위의 두가지의 방법론상 공통 특징
1> hand-crafted features
look-alike model은 사람이 수작업으로 만든 feature에 의존
collaborative filtering도 interaction matrix와 목적함수에 대한 design decision이 중요
이런 수기 feature engineering은 한계가 많다 (도메인 지식이 필요하거나, 테스트에 시간이 많이 거리거나, 계속 업데이트하고 관리해줘야 하는 것 등등)
그리고 model scalability의 한계 (모델이 자동 생성되고 유지되지 않으니까)
특히 데이터가 정형화된 소스가 아니라 준정형/비정형(로그 같은) 데이터로부터 나올 경우
2> aggregation of event sequences
전통적 ML 방법론은 fixed-lenth input feature vector를 필요로 함
그러나 고객 행동은 a sequence of events(페이지뷰, 클릭, 구매 등)인데, 이런건 aggreated features(average or total) 만들기가 어려움
그러다보니 lose information about temporal dependencies between events.
3> disconnect from content data
다양한 데이터 종류를 활용(행동, 텍스트, 이미지, 음성, 그래프 등)deep learning allows for more efficient personalization model architectures that derive and combine embeddings from behavioral, textual, and image data in a unified way
4> reliance on historical data
미래를 예측하기 위해서는, 과거 데이터를 활용하여 패턴을 학습한 relatively static environment를 가정한 것
5> focus on myopic optimization
전통적 개인화 모델은 designed to optimize an immediate action and its outcome (e.x. banner maximizes the click-through rate)
그러나 이제는 jointly optimize the sequence of actions( recommendations, offers or notifications)
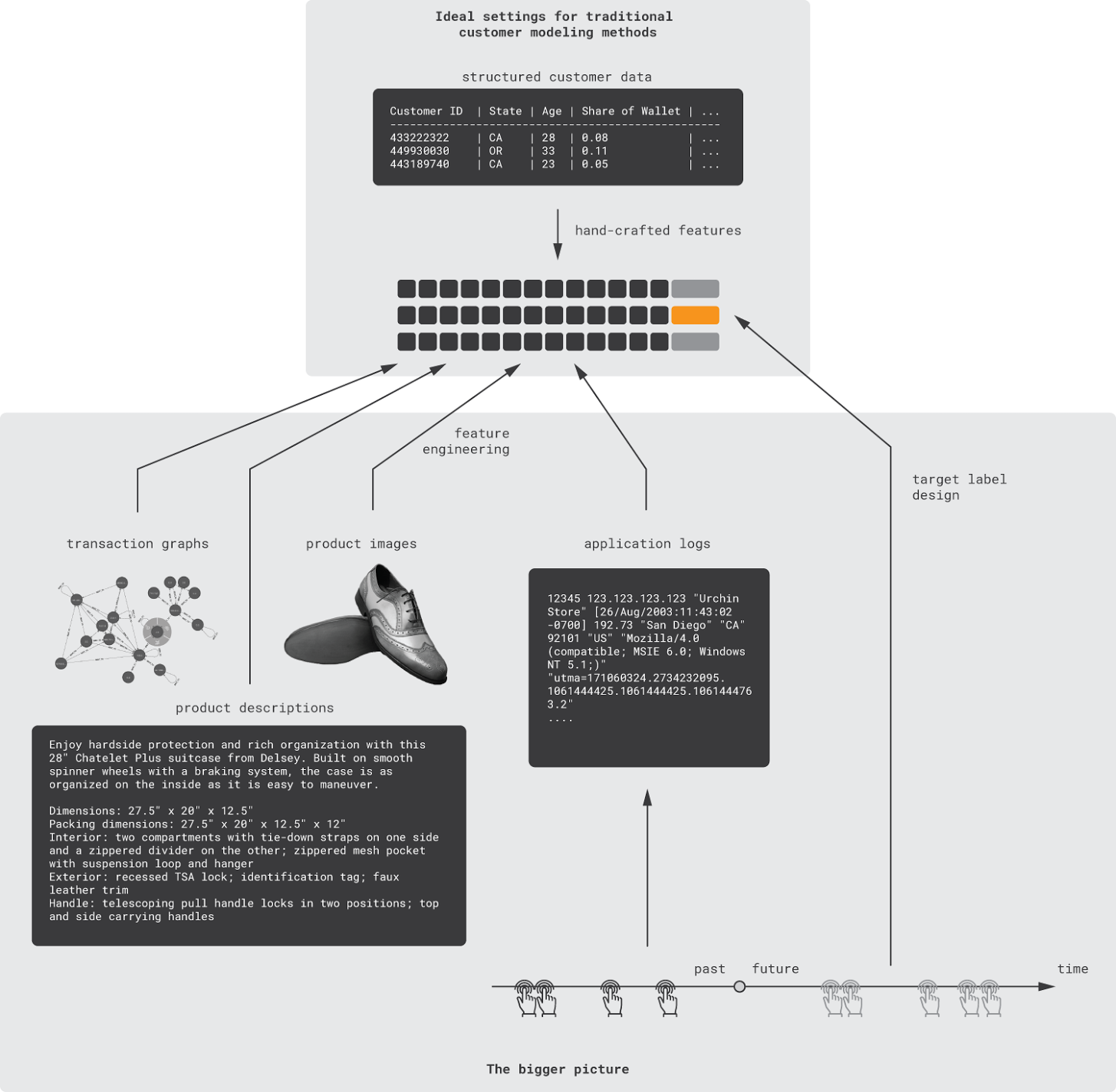
content-based filtering
demographic segmentation
demographic attributes and hand-crafted behavioral features
기존 방식의 한계를 극복하기 위한 방안
manually designed feature space / aggregated events / content data의 미비
-> 하단 참조
relience of history data / optimization
-> 강화학습
client2vec을 위한 Theory and Model
1 Learn from Event Sequences using RNNs
모델) LSTM, GRU
특징) 개별 이벤트를 recurrent neural network로 구성
함의) 고객 여정은 sequences of events 이며, each event is modeled as a vector of event-level features
(+) aggregated feature보다 이런 방식의 모델의 정확도가 더 높다
예시) churn prediction, conversion prediction, and product recommendation
(+) reduce complexity of feature engineering
by replacing customer-level features with event-level features
‘ it is much easier to design event features than design aggreates that summarize an entire customer history, including all important behavioral and demographic characteristics.’
(+) short event sequences 에 더 적합하다
예시) web session은 보통 2-3 가지의 이벤트로만 구성되어 있음
(+) hidden LSTM states를 customer embeddings으로 이해할 수 있다
예시) the values of individual elements of the state vector are traced over time, and these traces can be compared with the trace of the predicted metics - 여기에서 latent dimensions that are correlated with 를 찾아낼 수 있다
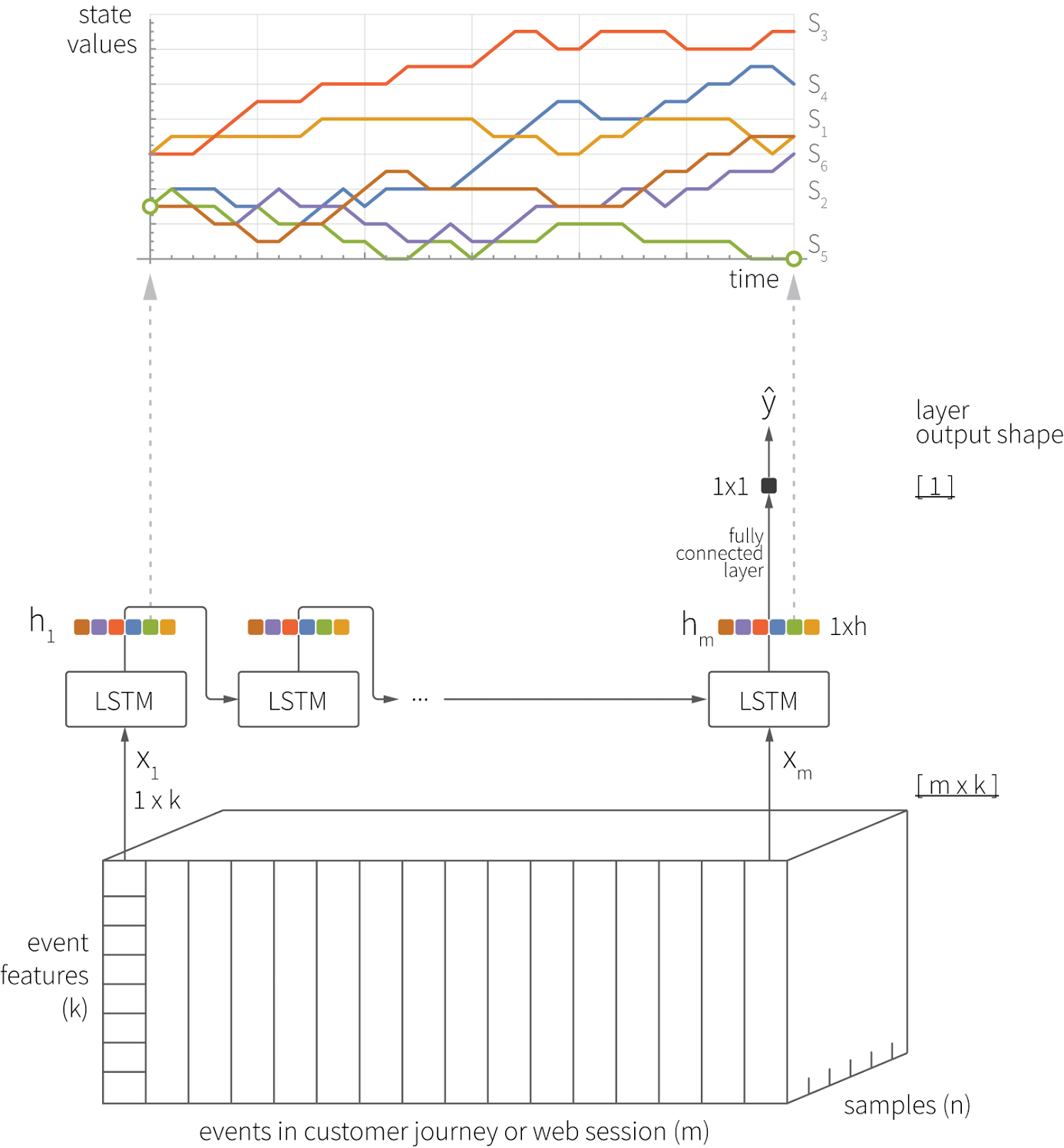
2 Learn Product Embeddings using NLP Methods
item2vec 을 구현하게 되면 의미있는 product embedding을 만들 수 있다
- 이를 통해 purchasing or browsing patterns 을 찾을 수 있고, 이는 products와 고객 사이의 affinity를 드러낸다
예시) canonical product categorizations - 음악으로 치자면 장르, 상품으로 치자면 카테고리
이거 다 비지도 학습 아닌가?
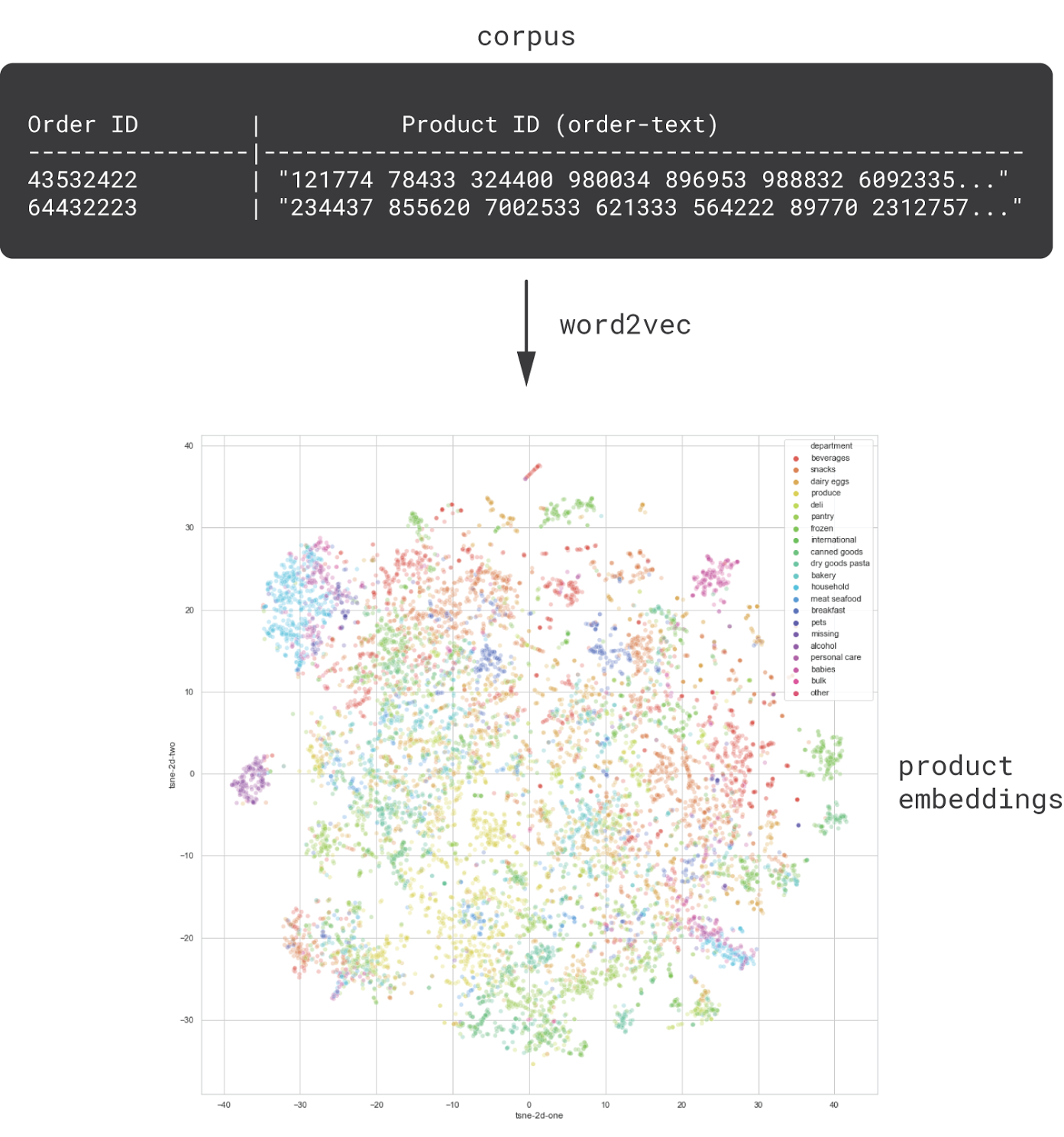
3 Mix Behavioral and Content Features
incorporate multiple heterogeneous data sources
예시) 주문정보와 web session을 flat sequences로 구성하여 attribute embedding을 학습시킬 수 있다
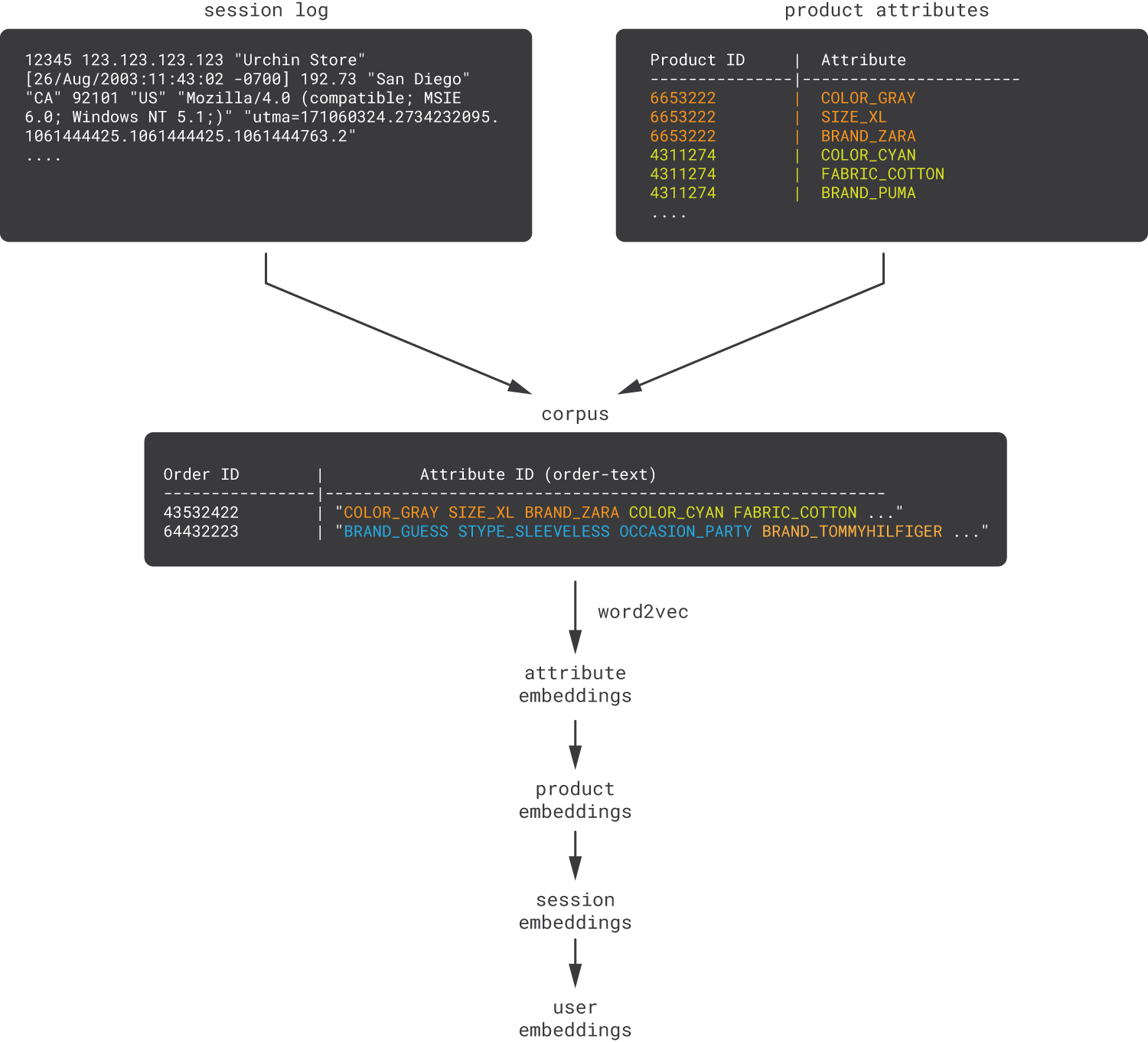
함의) purchasing patterns과 attribute-based product similaries를 동시에 알아낼 수 있다
-> 평균 내는 식의 roll-up 방식
함의) 구조화된 속성 정보가 없을 때도, 상품 정보를 사람이 읽을 수 있는 텍스트로 된 상품 속성 정보로 바꿀 수 있다
예시) item-to-item recommendation
user-to-item recommendation
contextual recommendation
automated feature engineering
product ID의 sequence 같은 정보를 활용하면 (item2vec 인가요), AutoML처럼 automated feature engineering을 고객 모델에 연계해서 활용할 수 있다
analytics and segmentation
기존 전통적인 hybrid recommendation algorithms에서 요구되는 섬세한 relevancy tuning이나 복잡한 feature engineering 필요 없다
4 Learn Customer Embeddings
한계) sequence를 반영할 뿐, hierarchical relationships를 표현하지 못한다
해결책) 문장을 임베딩하는 doc2vec 사용
응용) doc2vec으로 customer embedding을 한다는 것은?
customer sentences로부터 customer embedding 을 할 수 있다
예시) 머신러닝에서 텍스트로 future event에 대한 prediction이 가능하다
3번에서 기술한 produt embedding을 평균내서 customer embedding 하는 것으로는 이게 불가하다 ????? 이해가 안 되서 풀어써봄
corpus를 비교해보면 prod2vec에서는 영수증에 담긴 상품들(상품! 단어!)이 토크나이징 되어있었다면
여기에서는 상품이 아니라 고객에 대한 임베딩(상품들의 sequence! or action들의 문장!)이다 아래 그림 참조
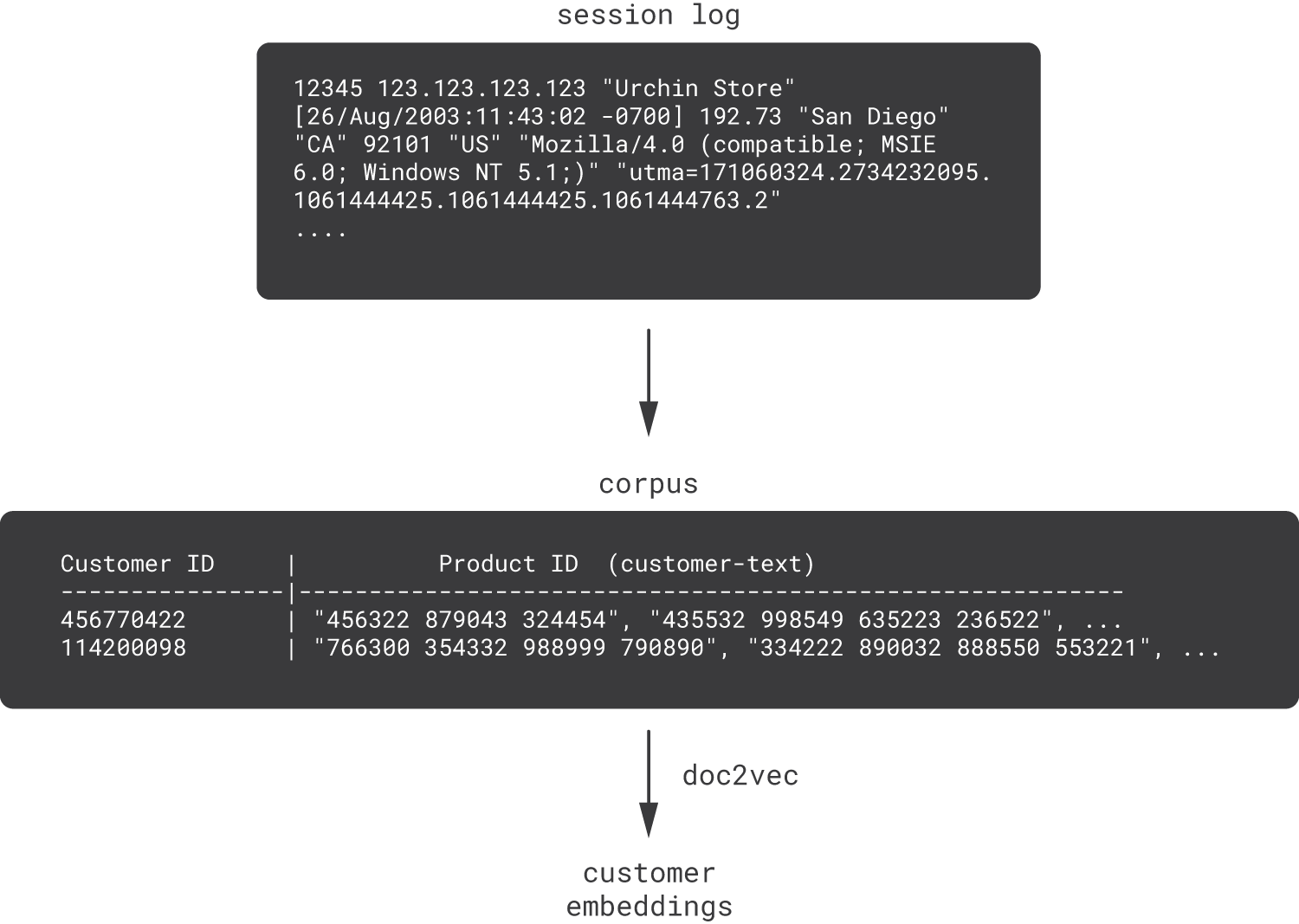
5 Learn Directly from Application Logs
특징) AutoML관점에서 customer/product/attributes와 같은 다른 레벨들의 aggregation별 embedding을 만들 뿐 아니라
bahavioral and content data를 섞는 것도 가능하다 행동! 컨텐츠!
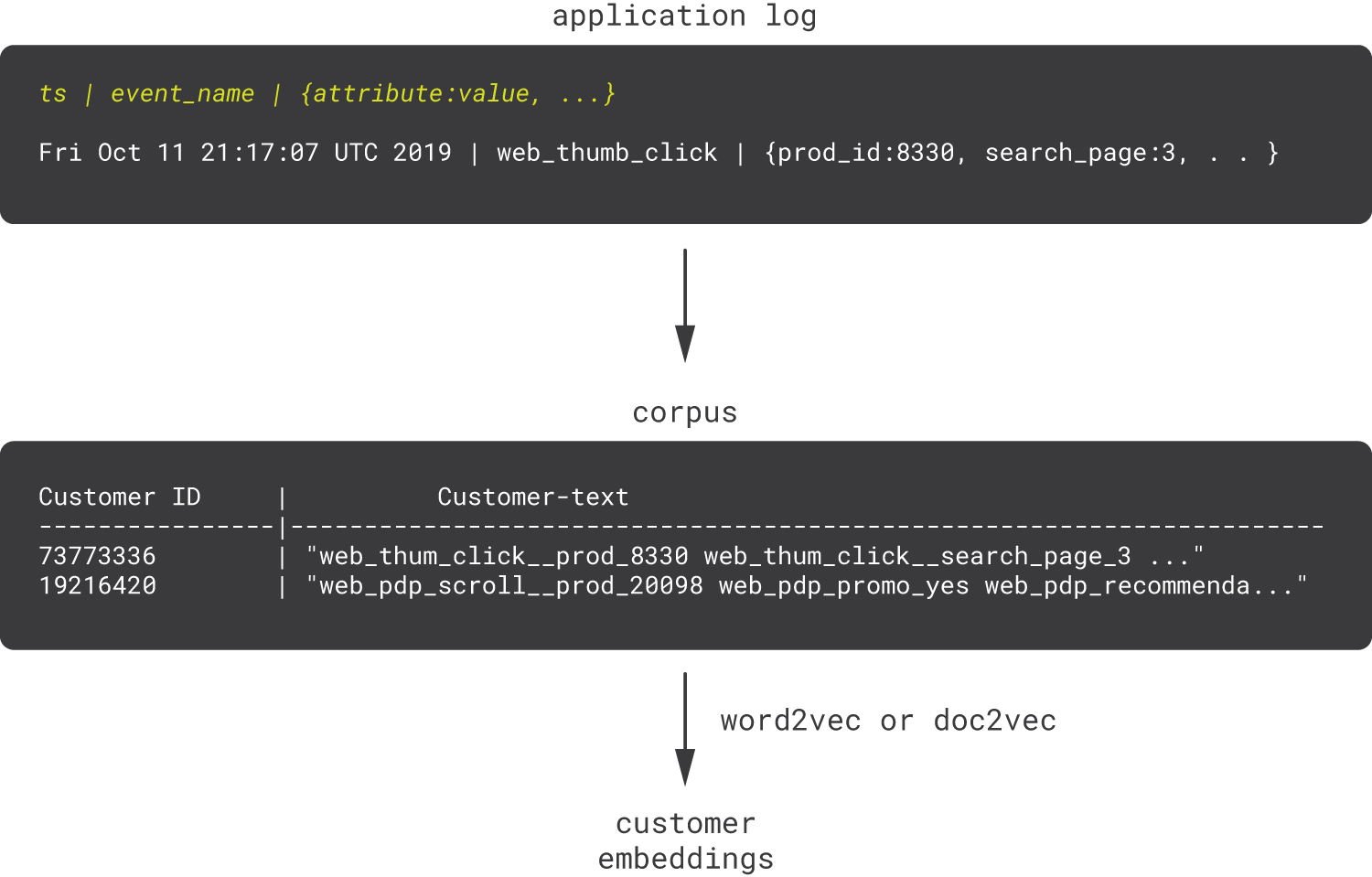
함의 ) AutoML관점에서, “customer-as-a-text paradigm”은 NLP를 도입한 고객분석을 가능케 하고, application log로부터 얻은 고객 데이터를 언어적으로 사용할 수 있게 한다
예시) conversion propensity 도출
price sensitivity scoring
예시) website or mobile application that continuously logs events with a timestamp, event type(click, view 등), event attribute(page, click coordinates)가 있다고 가정해보자
이걸 데이너사이언스가 수작업으로 feature engineering을 하는게 아니라
event names과 attributes를 discrete token분할된 토큰/분절된 토큰으로 ‘자동으로다가’ 연결해서 -> 토큰들이 customer-sentences를 구성하게 하면 된다.
token을 연결하면 customer-sentences가 되며, 여기에 customer2vec을 적용 할 수 있다
6 Guide the Search of Embeddings using Business Metrics
함의) downstream model 구조에서 자동화된 임베딩이 생성되게끔 하는 프로세스상에서
비즈니스 성과 지표를 학습해서 (this guided learning helps to align the latent space with business metrics)
학습한 embedding matrix를 활용하여
prediction을 푸는 문제에 적용할 수 있다
아래 그림에서 두 모델은 서로 독립적이나, 동시에 (별개로) 학습된다
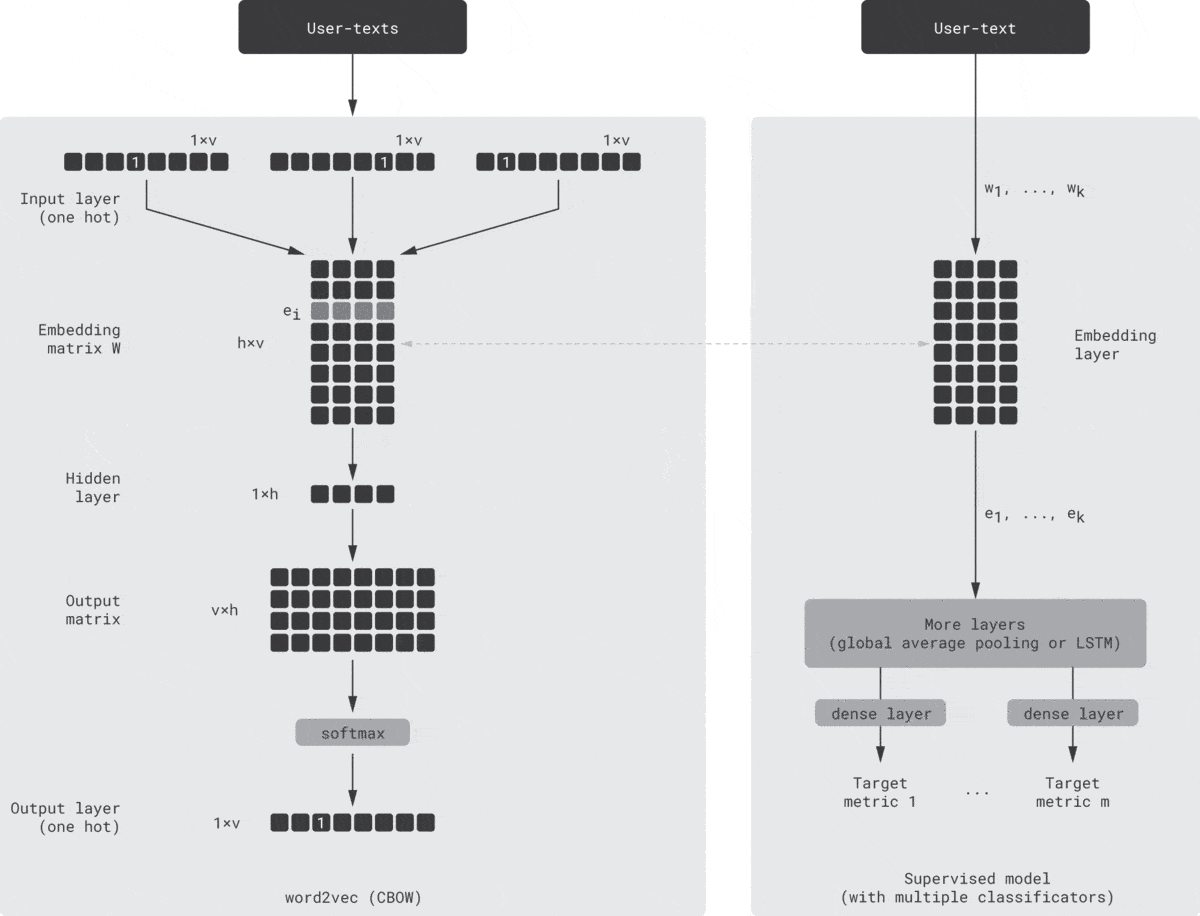
다시 서술하면, word2vec는 비지도 학습 방식의 모델이지만, supervised guidance을 주면서 이 모델은 enhancement 될 수 있다
아직도 다 이해가 안 된다
(오른쪽 모델) In the case of LSTM, we start with a supervised model but extract embeddings from the hidden layer.
이 모델은 several metrics를 hidden layer를 거쳐서 예측해내는 mult-output LSTM인데
이 hidden layer는 makes the LSTM schema even more similar to the guided word2vec model 로 만든다
7 Learn with Autoencoders
실은 NLP 모델은 꽤 복잡한데 (e.g. ELMo, BERT) 이를 개인화나 추천 모델에 적용하려면
autoencoder-based customer2vec model 도 하나의 방법
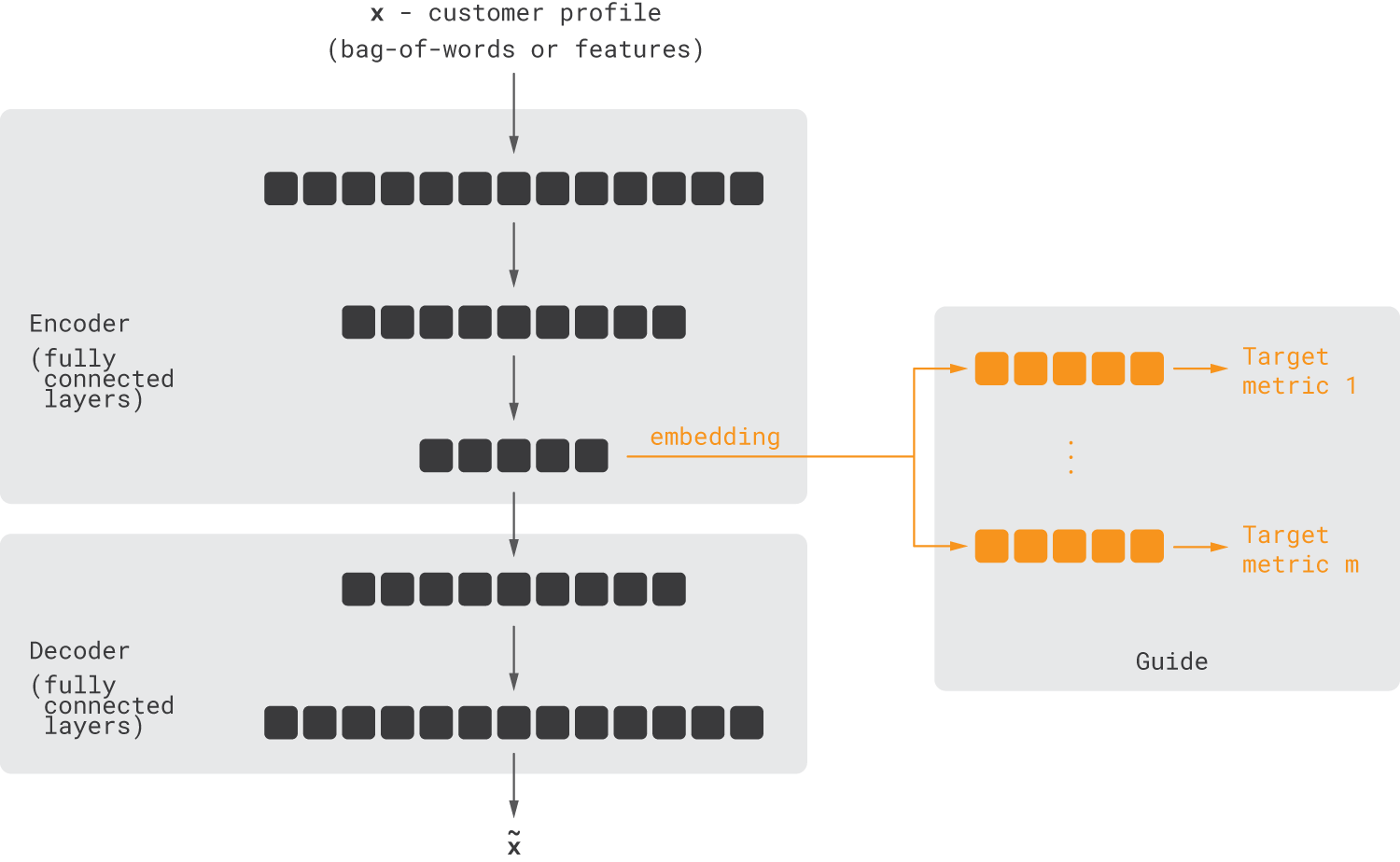
개념적으로,
input = fixed-length customer representation (= 수기로 만든 feature의 vector 이거나, customer-text로부터 생성한 BOW vector 일 수 있음)
encoder = 1 or several개의 신경망을 통해서 condensed representation 으로 축약 ( = customer embedding)
이때의 목적함수 = reconstruction error를 최소화하는 네트워크로 학습됨.
한 발 더 나아가서, weighted sum of the reconstruction loss 를 사용해서 전체적인 손실 함수를 최소화하도록 metrics를 guiding할 수 있다
decoder
output = expected values of the customer
8 graph, text, image 데이터의 통합
예시1) 금융 거래
예시2) 영상 추천
'algorithm > Deep Learning' 카테고리의 다른 글
NLP 6 - NLP 와 딥모델 (0) | 2021.09.05 |
---|---|
NLP 5 - 단어 임베딩 2 - word2vec (skip-gram 위주) (0) | 2021.08.01 |
NLP 4 - 단어 임베딩 (0) | 2021.08.01 |
중요!! 한국어 NLP 오픈소스 KLUE (1) | 2021.07.21 |
순환 신경망 RNN 1 (0) | 2021.07.05 |